Everything will be impacted by AI. Everything must be reviewed in the light of AI!
Entrepreneurs, managers, professionals, and all types of workers, including students are often alone and in full FOMO (Fear Of Missing Out)!
The vision, also shared by me, that in 2030 there will be no companies without AI at the center of the processes, is generating a lot of anxiety in managers, entrepreneurs, professionals who feel the need not to fall behind.
The mantra is to adopt anything as long as it's AI. As long as you lead your company to have an AI CORE.
And it's all urgent. Really?
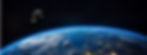
The web is full of experts (and gurus) who provide instant solutions to every problem, who provide tricks, ready prompt lists and as always the best application that you can start using right away.
But we know well that complexity has its times and its rules, that a new technology takes time to be applied, that improvising doesn't produce necessarily useful results.
And with AIs there is a risk of improvised, ill-reasoned choices, which lead to the introduction of unprepared oracles into the company to which one aspires to delegate the solution of each process, even the least suitable, forgetting that they cannot provide 100% reliability for their very nature.
So what should we do?
Obviously there is no universal answer.
I think the first thing to do is to understand more deeply what is happening, and what you need to do to prepare.
With this post I will begin to enter the corporate world a little more with Gen AI, after having directly experienced them on several occasions.
I won't be able to cover all of the topics and I expect this article to change over time as things continue to evolve. But the goal is to condense a little knowledge to allow you to decide better.
What are Foundation Models?
In the chaos of apps that all look the same, it is important to understand that they all start from the same foundations.
Foundation Models are AI based on deep learning models, pre-trained on very large amounts of data from very heterogeneous sources. They therefore include both algorithms, parameters and data. This allows them to gain a general understanding of the structures and patterns in the data, such as the grammar in a text or the characteristics of an image.
Once trained, they don't change anymore but they will be able to work on new contents that we provide them by comparing them to what they already know.
Then:
they don't know the color variants of your XYZ product, unless they were trained on that content (which maybe was public on your website before their training),
They need you to explain who you are every time you talk to them,
they don't remember your conversations nor do they use other people's conversations to look for answers to give to you.
The most famous are:
GPT 3.5 and 4.0 by OpenAI (here you will find an article explaining how it works) exposed in Chat GPT and Bing Chat
Claude.AI from Anthropic
Gemini on the chat interface of Google Bard
But the list is growing every day, open source models such as LLaMa 2, Falcon and thousands of others are being born. Which I have already talked about and will talk about in the blog.
How can we use them?
We know them for having used them through interfaces such as Chats but, when we talk about business use, it is important to point out that the most important ones provide access through different methods.
It is therefore important to consider that, in the company, they can be used in different ways:
Via Chat Chat GPT, Claude, Bard, Bing have accustomed us to a Chat system in which we insert more or less structured prompts, copy-paste a lot of information and press enter hoping to enter into an interesting conversation, through an interface that it is typically provided to us by the manufacturer of the chosen AI model. Remaining succumbed to the general conditions that often indicate that the data provided will be used by them to train new models (and not the one you are using at that moment). They often offer features that are only available this way. For example Open AI Provides an interface that opens to third-party plug-ins or code-interpreters which, for now, are only available in that mode.
Specialized Solutions and Plug-Ins “You'll stop using Chat GPT after you'll install this extension!” It is one of the many marketing messages found on THOUSANDS of small producers who develop alternative User Interfaces to that of Chat GPT to integrate them into browsers, search engines, pre-existing software that add more or less useful AI features to their own functionality . Microsoft's Copilot is one example, but there are hundreds of browser extensions made by small, up-and-coming companies. This involves the appearance of a new intermediate player between you and the physical executor of the processing. Who will obtain your data and, in "Black Box" mode, will forward them to the AI chosen by him (or by you).
Using via API. APIs (Application Programming Interfaces) are non-graphical but application interfaces that expose functions and procedures of an existing system. They therefore allow you to make calls between different applications either directly or through integration platforms. OPENAI was the first to release its API to allow you to integrate your existing solutions and add AI features that are much more 'under control' than previous solutions. And also by providing data protection policies that ensure that your data does not wander into the cloud unchecked.
Fine Tuning & RAG. Using the APIs it is possible to fine-tune the models, i.e. "integrate" them with a series of Questions and Answers based on your static datasets so that they always remember who you are, what you do, where you go. it is also possible to develop hybrid solutions of RAG (Retrieval-Augmented Generation) or integrate a 'search engine' to the LLM to obtain fresh data automatically in the prompt (Purists forgive me for my simplifications!)
Using Private templates. As I mentioned above, new more 'portable' models are emerging, able to be extremely customized for specific purposes with fine-tuning and other techniques that will make them much more suitable for your reality. I cite for example LLaMa 2 and Falcon but the list it is very long and growing. The advantages? Data security and control, Lock-in reduction with big players, cost control and above all extreme customization. On the other hand, they require internal technical skills and high design costs.

Understanding this is an essential step before thinking about 'Hiring an AI in the company'. But first of all it is important to give him a role.
But who (or rather: what) are you thinking of hiring?
A Co-Pilot, an Assistant, a CEO?
The best example, provided me Aditya Paul Berlia, Indian entrepreneur and 'AI Influencer' with whom, together with a large group of entrepreneurs, we are facing the evolution of Gen AI.
During a great AI workshop, organized with YPO, he said the following:
“Imagine, with AIs, that you have hired an incredibly talented new intern who has degrees from both Harvard and Stanford in numerous humanities and technical disciplines and all the best you can aspire. This intern knows just about everything about any subject you can imagine. However, he knows nothing about your specific company. He can't do anything if you don't first tell him who you are, what your goals are, how you want to get there.
Having state-of-the-art artificial intelligence at your disposal is exactly that: a very good intern with a vast knowledge who can provide you with answers on almost any topic, works 24/7, does not complain. But to get the most out of him in relation to your business, you'll need to train him and teach him all about your business. If you don't, his answers will always be based on general theories and not on information specific to your company. And they won't do you any good."
It is a guideline that I suggest to all entrepreneurs, professionals and managers, to start dealing with Gen AI: we can therefore think of having an extremely advanced personal assistant who is always available and who can collaborate with all our colleagues at every level. Reminding us that the responsibility for the choices and contents remains ours.
The worst AI available
During the same workshop I recorded another sentence of Adi to always keep in mind:
“Today we are using the worst version of Gen AI possible. The best is yet to come.”
And the advice is to keep the same approach in your company: it's an extremely fluid and rapidly changing world and, as often happens with technology, what is fundamental today becomes wrong (and harmful) tomorrow.
So it's good to set expectations. In these and in the coming months we will be bombarded with fantastic AI ideas and applications that we will want to adopt immediately. But discipline and method are needed. To ask ourselves what we really need and to fully understand the potential of these tools.
FOMO doesn't pay.
New approaches in the company: useful actions.
So let's try to understand how we should prepare for the arrival of an AI in the company.
1. Roles in the Company
The first thing that comes to mind is to appoint the AI manager in the company.
But, never as in this historical moment, it is a question of a non-choice.
The head of AI must be the CEO, or whoever has the complete vision of the company.
Re-read the sentence above because it is important.
With all due respect to the IT Managers and CTOs who will surely have to take care of implementing it, I believe that the CEO must fully understand the implications of this technology. He is dealing with a new type of resource and needs to study it and get his hands dirty firsthand. A bit like when PCs came out and we started talking about Hardware and Software for the first time.
And I'm not just talking about LLM (Multimodal, therefore from text, to images, to sound) but also, at least, hints of Machine Learning, Neural Networks, Deep Learning.
It is useful to know how to distinguish the different skills of the LLMs: NLU (Natural Language Understanding), NLG (Natural Language Generation), Question Answering, Summarization, Translation, Sentiment Analysis, Code Generation (!), Conversational Agents, Content Completion, Tutoring , Pattern Recognition and their ability to simulate characters and roles as well as having a clear understanding of the methods of adoption seen above.
But CEOs are sometimes too visionary even compared to their first level of management because they travel too far ahead. And if they are too fast, they risk leaving someone too far behind. This is why the staff must in turn be trained and made responsible, regardless of their role or technological skills.
Therefore, various "AI Advisors" must be defined. That is, the managers of the various departments and divisions who must, in turn, firsthand, understand what is happening out there and pass it on to their team. When fully operational, they will have to make sure that each department is making the most of AI to do its job.
Entrepreneurs, managers, professionals must UNDERSTAND AI, the technology behind it, before guiding the 'technicians'.
Later, in several months, we can talk about delegating this role.
The world has changed, again.
Note
2. Co-design of AI Systems:
Working with multidisciplinary teams is essential to create innovative and robust AI solutions. The diversity of skills and perspectives can lead to surprising results. Especially when everyone is aware of the possibilities available to them from technology.
So no more "technicians driven projects".
3. Agile Approach with Iterative Loops:
Agility in the process of development, testing and continuous feedback from users is essential. It is a method that allows you to quickly adapt to the ever-changing needs of the market. The term Agile is abused and has been talked about too much in recent years but when properly applied it can lead to extremely interesting results. In AI it is, in my opinion, essential.
4. Gradual Experimentation and Deployment:
Before a full deployment, it is wise to experiment and gradually implement AI solutions.
This allows you to detect and correct any problems at an early stage.
Small steps, prototypes and small results are a great start.
Solving small problems first and reviewing entire processes or business models later, when the team is aligned and the direction is understood.
5. Interoperability and integration:
Making sure AI systems can communicate and work with other systems is vital. Seamless integration can improve the efficiency and effectiveness of AI solutions across your organization. Today there are tons of integration solutions that are very simple to use to generate extremely robust and at the same time flexible and innovative workflows.
And if you remember: you are using the worst version of AI available today so you will need to change it quickly at some point.
6. Transparent Documentation:
Transparency in performance metrics, data used and logic implemented is essential. It provides a clear understanding of how AI systems work and helps build trust.
It's such an obvious concept that it's not valued as often as it's important.
7. AI Governance Framework:
Managing ethical, legal, and regulatory compliance issues is critical. A robust governance framework helps navigate this complex landscape, ensuring AI solutions are aligned with values and laws. Another huge theme that I won't deal with here.
8. Security and Data Protection:
Last but only to give it more weight. It goes without saying that data security and the protection of sensitive information are paramount.
By implementing AI, we are opening up our sensitive data or that of our stakeholders to companies that are often newborns or with questionable security and protection policies on data processing.
Let's always ask ourselves what is the business model of yet another supplier who offers amazing solutions at a low price. Often this happens because their possession of the data is worth much more than the negligible monthly fee they ask you.
It is also essential to give the right weight to each area: it makes no sense to protect data with Fort Knox that normally circulate in the company on the various cloud drives or in the emails of each collaborator.
Often when I talk to entrepreneurs and managers I ask them, based on what they've heard, what expectations they have from Gen AI. Sometimes I hear embarrassing ones for how much they underestimate the problems underlying their implementation. It is true that everything seems very simple, but scaling expectations is very useful, as well as to avoid disappointments, also to reduce the probability of making wrong investments.
Correct expectations
✅ The results must be 'good enough', not perfect. The Foundation Models, as we have said, are static models that use new information, compare it to what they have learned, and TRY to give answers that make sense to us. Most of the time they succeed very well, especially if the prompts are adequate (here my contribution on expectations in conversations with AI). But they don't guarantee us 100% perfection and absence of hallucinations. If you are looking for 100%, get ready for different investments or, at least, to use more traditional technologies together with AI.
✅ Gen AIs need to reduce your costs or increase your earnings. Otherwise, I'm wasted time. And I don't feel like extending myself further on this very clear topic. But AIs can generate a lot of vanity metrics: information that is great to highlight to show that results are being achieved but are not correlated with cost or revenue trends or, more often, are falsely correlated.
✅ Magic and instantaneousness DO NOT exist. It takes time to get things done. The state of the art of the LLMs, of the integration systems and the ease of developing "AI Centric" interfaces allow today to create wonderful amusement parks in a very short time. But bringing new processes into production is never free, it doesn't happen too quickly.Only two can be optimized between implementation time, quality of results, implementation cost.I borrowed this saying that has been around for quite some time in the consulting world. Read it again and prove to me that it is not true: I am ready to change my mind. 😊
✅ AI is a tool, not an oracle or an almighty god. We are talking about software, highly evolved, wonderful, easy to learn (to start with) but subject to the same problems as any other tool based on data and algorithms that you adopt in the company. The steps to take are always the same: from the selection of the right tool, to its implementation in the company, to the training of people starting from the search for the processes that most deserve it.
✅ The one in circulation is the worst version of AI available. Repetita iuvant. There has never been a time like this when each 'application' has so many 'solutions' available, so many different APPs, to achieve the same result. And the behind-the-scenes work that many companies are doing is leading to better and better versions of each solution. So don't fixate on a technology or a company that seems the best today: it will change tomorrow. And in your integration you will have to take this into account by using several at the same time and letting the best ones win over time.
Wrong expectations
❌ Who has a global vision of things. May she knows everything. Models know the data they were trained on plus any Fine Tuning or embedding (which I realize I'll have to detail shortly) plus the little context you provide them in the prompt. We are talking today between 4k and 100k tokens.Which are not few (The Divine Comedy has about 101,000 characters) but not even very many when it comes to solving complicated problems or very dirty starting data. Calculate that each token corresponds to about 5-6 characters to do the calculations.
❌ That it is very simple to implement it quickly and correctly. See all points above. It is very easy to leave, more difficult to maintain a course in this storm of new announcements and daily contradictions.
❌ May she take the initiative on her own and solve all your problems. AI has no ends but yours. It has no goals other than the specific ones that you give it.We are working on autonomous agents, of course, but every initiative in the company starts from you, your collaborators or one of your human stakeholders and ends when this is satisfied.Not even Gen AI can have a goal like "Keep the company in order, make it grow and prosper, solve the problems that will come!". We would need an AI hired as CEO. But we'll talk about it in a few years.
Note:However, this prompt “Keep the company in order, make it grow and prosper, solve the problems that will come!” scares me a lot, because it contains no constraints. Feed it to a General Artificial Intelligence, if you know Bolstrom's paper clip theory, would perhaps bring about the end of the world.
❌ That it is advanced enough to face all problems. I trivialize by saying that we cannot think of developing an ERP with AI. Although it will surely be able to use your developers to create new ERP software in a more advanced way. But it can't solve every dark corner of your company. It should be applied, for now, only to some areas and for certain purposes. I will talk about it at length in the next post.
❌May he clean your "dirty data" "soon and well The saying "Garbage In Garbage Out" remains valid in the world of LLMs. If you feed dirty data to an algorithm you will get dirty results. And then:
Dirty Data > Poor AI Results > Poor Business Results.
AI can do a great job to clean some datasets, classify them, normalize them but often it won't be enough.
And speaking of that:
Do you have full ownership of your data?
I mean: does every system adopted in the company, every process you run, basically every software, provide you with updated data in real time?
Do you own this information or is your supplier reticent?
If you don't have this information, feel free to threaten him and tell him you don't trust him.
And in any case by data I don't mean only PDFs, eMails, documents, spreadsheets and presentations but structured data available to be fed to GEN AI to obtain correct information and reduce hallucinations.
We need structured, clean, accessible data.
Also, data is of little use if you don't know your processes.
Do you know your processes?
I mean: are they documented, clear, updated? Do they exist on paper/pdf sheets or are they structured on electronic documents or managed by workflow software?
Without understanding which processes are running in the company, without getting angry because they are not sufficiently documented, updated, accessible, it will be difficult to understand how to trigger AI in processes that reduce costs or increase revenues.
I'll stop here for now. I know your attention has limits (Mine definitely more) and right now you have important things to do 🙂.
However, I'm very curious to know what you think, write to me or comment because in the next post we'll go into much more practicality to try to understand the steps to take.
Here is a glossary of the 'Too AI' terms used in the post.
As always, I invite you to reflect, to comment, to spread ideas by spreading this post with the people you think might be interested.
To stay updated on my content:
Read Glimpse, my AI novel (only in Italian).
Or contact me here.
See you next time!
Massimiliano Turazzini